Anit Kumar Sahu
PhD from CMU working in ML/AI
Research Expertise
About
Publications
Federated Learning: Challenges, Methods, and Future Directions
IEEE Signal Processing Magazine / May 01, 2020
Li, T., Sahu, A. K., Talwalkar, A., & Smith, V. (2020). Federated Learning: Challenges, Methods, and Future Directions. IEEE Signal Processing Magazine, 37(3), 50–60. https://doi.org/10.1109/msp.2020.2975749
FedDANE: A Federated Newton-Type Method
2019 53rd Asilomar Conference on Signals, Systems, and Computers / Nov 01, 2019
Li, T., Sahu, A. K., Zaheer, M., Sanjabi, M., Talwalkar, A., & Smithy, V. (2019, November). FedDANE: A Federated Newton-Type Method. 2019 53rd Asilomar Conference on Signals, Systems, and Computers. https://doi.org/10.1109/ieeeconf44664.2019.9049023
MATCHA: Speeding Up Decentralized SGD via Matching Decomposition Sampling
2019 Sixth Indian Control Conference (ICC) / Dec 01, 2019
Wang, J., Sahu, A. K., Yang, Z., Joshi, G., & Kar, S. (2019, December). MATCHA: Speeding Up Decentralized SGD via Matching Decomposition Sampling. 2019 Sixth Indian Control Conference (ICC). https://doi.org/10.1109/icc47138.2019.9123209
Learning representations in Bayesian Confidence Propagation neural networks
2020 International Joint Conference on Neural Networks (IJCNN) / Jul 01, 2020
Ravichandran, N. B., Lansner, A., & Herman, P. (2020, July). Learning representations in Bayesian Confidence Propagation neural networks. 2020 International Joint Conference on Neural Networks (IJCNN). https://doi.org/10.1109/ijcnn48605.2020.9207061
Distributed stochastic optimization with gradient tracking over strongly-connected networks
2019 IEEE 58th Conference on Decision and Control (CDC) / Dec 01, 2019
Xin, R., Sahu, A. K., Khan, U. A., & Kar, S. (2019, December). Distributed stochastic optimization with gradient tracking over strongly-connected networks. 2019 IEEE 58th Conference on Decision and Control (CDC). https://doi.org/10.1109/cdc40024.2019.9029217
Convergence Rates for Distributed Stochastic Optimization Over Random Networks
2018 IEEE Conference on Decision and Control (CDC) / Dec 01, 2018
Jakovetic, D., Bajovic, D., Sahu, A. K., & Kar, S. (2018, December). Convergence Rates for Distributed Stochastic Optimization Over Random Networks. 2018 IEEE Conference on Decision and Control (CDC). https://doi.org/10.1109/cdc.2018.8619228
Distributed Zeroth Order Optimization Over Random Networks: A Kiefer-Wolfowitz Stochastic Approximation Approach
2018 IEEE Conference on Decision and Control (CDC) / Dec 01, 2018
Kumar Sahu, A., Jakovetic, D., Bajovic, D., & Kar, S. (2018, December). Distributed Zeroth Order Optimization Over Random Networks: A Kiefer-Wolfowitz Stochastic Approximation Approach. 2018 IEEE Conference on Decision and Control (CDC). https://doi.org/10.1109/cdc.2018.8619044
Distributed Constrained Recursive Nonlinear Least-Squares Estimation: Algorithms and Asymptotics
IEEE Transactions on Signal and Information Processing over Networks / Jan 01, 2016
Sahu, A. K., Kar, S., Moura, J. M. F., & Poor, H. V. (2016). Distributed Constrained Recursive Nonlinear Least-Squares Estimation: Algorithms and Asymptotics. IEEE Transactions on Signal and Information Processing over Networks, 1–1. https://doi.org/10.1109/tsipn.2016.2618318
Federated Learning Challenges and Opportunities: An Outlook
ICASSP 2022 - 2022 IEEE International Conference on Acoustics, Speech and Signal Processing (ICASSP) / May 23, 2022
Ding, J., Tramel, E., Sahu, A. K., Wu, S., Avestimehr, S., & Zhang, T. (2022, May 23). Federated Learning Challenges and Opportunities: An Outlook. ICASSP 2022 - 2022 IEEE International Conference on Acoustics, Speech and Signal Processing (ICASSP). https://doi.org/10.1109/icassp43922.2022.9746925
Simple and Efficient Hard Label Black-box Adversarial Attacks in Low Query Budget Regimes
Proceedings of the 27th ACM SIGKDD Conference on Knowledge Discovery & Data Mining / Aug 14, 2021
Shukla, S. N., Sahu, A. K., Willmott, D., & Kolter, Z. (2021, August 14). Simple and Efficient Hard Label Black-box Adversarial Attacks in Low Query Budget Regimes. Proceedings of the 27th ACM SIGKDD Conference on Knowledge Discovery & Data Mining. https://doi.org/10.1145/3447548.3467386
About the social role of child and adolescent psychiatrists in times of epidemic
IACAPAP ArXiv / Jan 01, 2020
Falissard, B. (2020). About the social role of child and adolescent psychiatrists in times of epidemic. IACAPAP ArXiv. https://doi.org/10.14744/iacapaparxiv.2020.20004
Recursive Distributed Detection for Composite Hypothesis Testing: Nonlinear Observation Models in Additive Gaussian Noise
IEEE Transactions on Information Theory / Aug 01, 2017
Sahu, A. K., & Kar, S. (2017). Recursive Distributed Detection for Composite Hypothesis Testing: Nonlinear Observation Models in Additive Gaussian Noise. IEEE Transactions on Information Theory, 63(8), 4797–4828. https://doi.org/10.1109/tit.2017.2686435
NON-ASYMPTOTIC RATES FOR COMMUNICATION EFFICIENT DISTRIBUTED ZEROTH ORDER STRONGLY CONVEX OPTIMIZATION
2018 IEEE Global Conference on Signal and Information Processing (GlobalSIP) / Nov 01, 2018
Sahu, A. K., Jakovetic, D., Bajovic, D., & Kar, S. (2018, November). NON-ASYMPTOTIC RATES FOR COMMUNICATION EFFICIENT DISTRIBUTED ZEROTH ORDER STRONGLY CONVEX OPTIMIZATION. 2018 IEEE Global Conference on Signal and Information Processing (GlobalSIP). https://doi.org/10.1109/globalsip.2018.8646406
$\mathcal {CIRFE}$: A Distributed Random Fields Estimator
IEEE Transactions on Signal Processing / Sep 15, 2018
Sahu, A. K., Jakovetic, D., & Kar, S. (2018). $\mathcal {CIRFE}$: A Distributed Random Fields Estimator. IEEE Transactions on Signal Processing, 66(18), 4980–4995. https://doi.org/10.1109/tsp.2018.2863646
CREDO: A Communication-Efficient Distributed Estimation Algorithm
2018 IEEE International Symposium on Information Theory (ISIT) / Jun 01, 2018
Sahu, A. K., Jakovetic, D., & Kar, S. (2018, June). CREDO: A Communication-Efficient Distributed Estimation Algorithm. 2018 IEEE International Symposium on Information Theory (ISIT). https://doi.org/10.1109/isit.2018.8437640
ActPerFL: Active Personalized Federated Learning
Proceedings of the First Workshop on Federated Learning for Natural Language Processing (FL4NLP 2022) / Jan 01, 2022
Chen, H., Ding, J., Tramel, E., Wu, S., Sahu, A. K., Avestimehr, S., & Zhang, T. (2022). ActPerFL: Active Personalized Federated Learning. Proceedings of the First Workshop on Federated Learning for Natural Language Processing (FL4NLP 2022). https://doi.org/10.18653/v1/2022.fl4nlp-1.1
Decentralized Zeroth-Order Constrained Stochastic Optimization Algorithms: Frank–Wolfe and Variants With Applications to Black-Box Adversarial Attacks
Proceedings of the IEEE / Nov 01, 2020
Sahu, A. K., & Kar, S. (2020). Decentralized Zeroth-Order Constrained Stochastic Optimization Algorithms: Frank–Wolfe and Variants With Applications to Black-Box Adversarial Attacks. Proceedings of the IEEE, 108(11), 1890–1905. https://doi.org/10.1109/jproc.2020.3012609
Data-driven Thermal Model Inference with ARMAX, in Smart Environments, based on Normalized Mutual Information
2018 Annual American Control Conference (ACC) / Jun 01, 2018
Jiang, Z., Francis, J., Sahu, A. K., Munir, S., Shelton, C., Rowe, A., & Berges, M. (2018, June). Data-driven Thermal Model Inference with ARMAX, in Smart Environments, based on Normalized Mutual Information. 2018 Annual American Control Conference (ACC). https://doi.org/10.23919/acc.2018.8431085
Partial model averaging in Federated Learning: Performance guarantees and benefits
Neurocomputing / Nov 01, 2023
Lee, S., Sahu, A. K., He, C., & Avestimehr, S. (2023). Partial model averaging in Federated Learning: Performance guarantees and benefits. Neurocomputing, 556, 126647. https://doi.org/10.1016/j.neucom.2023.126647
Nonlinear Gradient Mappings and Stochastic Optimization: A General Framework with Applications to Heavy-Tail Noise
SIAM Journal on Optimization / May 16, 2023
Jakovetić, D., Bajović, D., Sahu, A. K., Kar, S., Milos̆ević, N., & Stamenković, D. (2023). Nonlinear Gradient Mappings and Stochastic Optimization: A General Framework with Applications to Heavy-Tail Noise. SIAM Journal on Optimization, 33(2), 394–423. https://doi.org/10.1137/21m145896x
Matcha: A Matching-Based Link Scheduling Strategy to Speed up Distributed Optimization
IEEE Transactions on Signal Processing / Jan 01, 2022
Wang, J., Sahu, A. K., Joshi, G., & Kar, S. (2022). Matcha: A Matching-Based Link Scheduling Strategy to Speed up Distributed Optimization. IEEE Transactions on Signal Processing, 70, 5208–5221. https://doi.org/10.1109/tsp.2022.3212536
Communication efficient distributed weighted non-linear least squares estimation
EURASIP Journal on Advances in Signal Processing / Oct 19, 2018
Sahu, A. K., Jakovetic, D., Bajovic, D., & Kar, S. (2018). Communication efficient distributed weighted non-linear least squares estimation. EURASIP Journal on Advances in Signal Processing, 2018(1). https://doi.org/10.1186/s13634-018-0586-0
ILASR: Privacy-Preserving Incremental Learning for Automatic Speech Recognition at Production Scale
Proceedings of the 28th ACM SIGKDD Conference on Knowledge Discovery and Data Mining / Aug 14, 2022
Chennupati, G., Rao, M., Chadha, G., Eakin, A., Raju, A., Tiwari, G., Sahu, A. K., Rastrow, A., Droppo, J., Oberlin, A., Nandanoor, B., Venkataramanan, P., Wu, Z., & Sitpure, P. (2022, August 14). ILASR: Privacy-Preserving Incremental Learning for Automatic Speech Recognition at Production Scale. Proceedings of the 28th ACM SIGKDD Conference on Knowledge Discovery and Data Mining. https://doi.org/10.1145/3534678.3539174
Dist-Hedge: A partial information setting based distributed non-stochastic sequence prediction algorithm
2017 IEEE Global Conference on Signal and Information Processing (GlobalSIP) / Nov 01, 2017
Sahu, A. K., & Kar, S. (2017, November). Dist-Hedge: A partial information setting based distributed non-stochastic sequence prediction algorithm. 2017 IEEE Global Conference on Signal and Information Processing (GlobalSIP). https://doi.org/10.1109/globalsip.2017.8308699
Exploring the Error-Runtime Trade-off in Decentralized Optimization
2020 54th Asilomar Conference on Signals, Systems, and Computers / Nov 01, 2020
Wang, J., Sahu, A. K., Joshi, G., & Kar, S. (2020, November 1). Exploring the Error-Runtime Trade-off in Decentralized Optimization. 2020 54th Asilomar Conference on Signals, Systems, and Computers. https://doi.org/10.1109/ieeeconf51394.2020.9443529
Deep Active Learning with Noisy Oracle in Object Detection
Proceedings of the 19th International Joint Conference on Computer Vision, Imaging and Computer Graphics Theory and Applications / Jan 01, 2024
Schubert, M., Riedlinger, T., Kahl, K., & Rottmann, M. (2024). Deep Active Learning with Noisy Oracle in Object Detection. Proceedings of the 19th International Joint Conference on Computer Vision, Imaging and Computer Graphics Theory and Applications. https://doi.org/10.5220/0012315800003660
Communication Efficient Distributed Estimation Over Directed Random Graphs
IEEE EUROCON 2019 -18th International Conference on Smart Technologies / Jul 01, 2019
Sahu, A. K., Jakovetic, D., Bajovic, D., & Kar, S. (2019, July). Communication Efficient Distributed Estimation Over Directed Random Graphs. IEEE EUROCON 2019 -18th International Conference on Smart Technologies. https://doi.org/10.1109/eurocon.2019.8861544
Field performance analysis of solar cell designs
Journal of Power Sources Advances / Apr 01, 2024
Hwang, S., Suh, D., & Kang, Y. (2024). Field performance analysis of solar cell designs. Journal of Power Sources Advances, 26, 100145. https://doi.org/10.1016/j.powera.2024.100145
Federated Self-Learning with Weak Supervision for Speech Recognition
ICASSP 2023 - 2023 IEEE International Conference on Acoustics, Speech and Signal Processing (ICASSP) / Jun 04, 2023
Rao, M., Chennupati, G., Tiwari, G., Kumar Sahu, A., Raju, A., Rastrow, A., & Droppo, J. (2023, June 4). Federated Self-Learning with Weak Supervision for Speech Recognition. ICASSP 2023 - 2023 IEEE International Conference on Acoustics, Speech and Signal Processing (ICASSP). https://doi.org/10.1109/icassp49357.2023.10096983
Distributed empirical risk minimization over directed graphs
2019 53rd Asilomar Conference on Signals, Systems, and Computers / Nov 01, 2019
Xin, R., Sahu, A. K., Kar, S., & Khan, U. A. (2019, November). Distributed empirical risk minimization over directed graphs. 2019 53rd Asilomar Conference on Signals, Systems, and Computers. https://doi.org/10.1109/ieeeconf44664.2019.9049065
arXiv
100 Years of Math Milestones / Jun 12, 2019
arXiv. (2019). In 100 Years of Math Milestones (pp. 433–437). American Mathematical Society. https://doi.org/10.1090/mbk/121/79
Large Deviations for Products of Non-Identically Distributed Network Matrices With Applications to Communication-Efficient Distributed Learning and Inference
IEEE Transactions on Signal Processing / Jan 01, 2023
Petrović, N., Bajović, D., Kar, S., Jakovetić, D., & Sahu, A. K. (2023). Large Deviations for Products of Non-Identically Distributed Network Matrices With Applications to Communication-Efficient Distributed Learning and Inference. IEEE Transactions on Signal Processing, 71, 1319–1333. https://doi.org/10.1109/tsp.2023.3263254
Inertial Projection Method for Solving Monotone Operator Equations
2022 12th International Conference on Information Science and Technology (ICIST) / Oct 14, 2022
Abubakar, A. B., Feng, Y., & Ibrahim, A. H. (2022, October 14). Inertial Projection Method for Solving Monotone Operator Equations. 2022 12th International Conference on Information Science and Technology (ICIST). https://doi.org/10.1109/icist55546.2022.9926859
Scientometric engineering: Exploring citation dynamics via arXiv eprints
Quantitative Science Studies / Jan 01, 2022
Okamura, K. (2022). Scientometric engineering: Exploring citation dynamics via arXiv eprints. Quantitative Science Studies, 3(1), 122–146. https://doi.org/10.1162/qss_a_00174
Large Deviations for Products of Non-I.i.d. Stochastic Matrices with Application to Distributed Detection
2018 IEEE International Symposium on Information Theory (ISIT) / Jun 01, 2018
Bajovic, D., Jakovetic, D., Sahu, A. K., & Kar, S. (2018, June). Large Deviations for Products of Non-I.i.d. Stochastic Matrices with Application to Distributed Detection. 2018 IEEE International Symposium on Information Theory (ISIT). https://doi.org/10.1109/isit.2018.8437732
Distributed sequence prediction: A consensus+innovations approach
2016 IEEE Global Conference on Signal and Information Processing (GlobalSIP) / Dec 01, 2016
Sahu, A. K., & Kar, S. (2016, December). Distributed sequence prediction: A consensus+innovations approach. 2016 IEEE Global Conference on Signal and Information Processing (GlobalSIP). https://doi.org/10.1109/globalsip.2016.7905854
Distributed generalized likelihood ratio tests: Fundamental limits and tradeoffs
2016 IEEE International Conference on Acoustics, Speech and Signal Processing (ICASSP) / Mar 01, 2016
Sahu, A. K., & Kar, S. (2016, March). Distributed generalized likelihood ratio tests: Fundamental limits and tradeoffs. 2016 IEEE International Conference on Acoustics, Speech and Signal Processing (ICASSP). https://doi.org/10.1109/icassp.2016.7472543
Distributed Recursive Estimation under Heavy-Tail Communication Noise
SIAM Journal on Control and Optimization / Jun 20, 2023
Jakovetic, D., Vukovic, M., Bajovic, D., Sahu, A. K., & Kar, S. (2023). Distributed Recursive Estimation under Heavy-Tail Communication Noise. SIAM Journal on Control and Optimization, 61(3), 1582–1609. https://doi.org/10.1137/22m1477015
Preprint repository arXiv achieves milestone million uploads
Physics Today / Jan 01, 2014
Preprint repository arXiv achieves milestone million uploads. (2014). Physics Today. https://doi.org/10.1063/pt.5.028530
Distributed recursive composite hypothesis testing: Imperfect communication
2016 IEEE International Symposium on Information Theory (ISIT) / Jul 01, 2016
Sahu, A. K., & Kar, S. (2016, July). Distributed recursive composite hypothesis testing: Imperfect communication. 2016 IEEE International Symposium on Information Theory (ISIT). https://doi.org/10.1109/isit.2016.7541785
On Arxiv Moderation System
Jan 01, 2023
Silagadze, Z. (2023). On Arxiv Moderation System. https://doi.org/10.2139/ssrn.4392249
Fine Tuning Auto Regressive LLMs for Long Document Abstractive Summarization
2023 IEEE 2nd International Conference on Industrial Electronics: Developments & Applications (ICIDeA) / Sep 29, 2023
Rath, M. K., Banerjee, S., & Swain, T. (2023, September 29). Fine Tuning Auto Regressive LLMs for Long Document Abstractive Summarization. 2023 IEEE 2nd International Conference on Industrial Electronics: Developments & Applications (ICIDeA). https://doi.org/10.1109/icidea59866.2023.10295238
Federated Representation Learning for Automatic Speech Recognition
3rd Symposium on Security and Privacy in Speech Communication / Aug 19, 2023
Ramesh, G. V., Chennupati, G., Rao, M., Sahu, A. K., Rastrow, A., & Droppo, J. (2023, August 19). Federated Representation Learning for Automatic Speech Recognition. 3rd Symposium on Security and Privacy in Speech Communication. https://doi.org/10.21437/spsc.2023-5
Code2Drive: A Code-based Interactive and Educational Driving Environment for Improving the Youth Driving Learning and Training using Machine Learning
Machine Learning & Applications / Jun 17, 2023
Lin, Z., & Sahagun, J. (2023, June 17). Code2Drive: A Code-based Interactive and Educational Driving Environment for Improving the Youth Driving Learning and Training using Machine Learning. Machine Learning & Applications. https://doi.org/10.5121/csit.2023.131014
What Learned Representations and Influence Functions Can Tell Us About Adversarial Examples
Findings of the Association for Computational Linguistics: IJCNLP-AACL 2023 (Findings) / Jan 01, 2023
Tonni, S. M., & Dras, M. (2023). What Learned Representations and Influence Functions Can Tell Us About Adversarial Examples. Findings of the Association for Computational Linguistics: IJCNLP-AACL 2023 (Findings). https://doi.org/10.18653/v1/2023.findings-ijcnlp.35
Learning When to Trust Which Teacher for Weakly Supervised ASR
INTERSPEECH 2023 / Aug 20, 2023
Agrawal, A., Rao, M., Sahu, A. K., Chennupati, G., & Stolcke, A. (2023, August 20). Learning When to Trust Which Teacher for Weakly Supervised ASR. INTERSPEECH 2023. https://doi.org/10.21437/interspeech.2023-2205
Token Selection from Multiple Input Places
Asset Analytics / Jan 01, 2023
Davidrajuh, R. (2023). Token Selection from Multiple Input Places. In Colored Petri Nets for Modeling of Discrete Systems (pp. 59–65). Springer Nature Singapore. https://doi.org/10.1007/978-981-99-6859-6_4
Velocimeter LIDAR-Based Multiplicative Extended Kalman Filter for Terrain Relative Navigation App...
Jan 03, 2022
Velocimeter LIDAR-Based Multiplicative Extended Kalman Filter for Terrain Relative Navigation App... (2022). American Institute of Aeronautics and Astronautics (AIAA). https://doi.org/10.2514/6.2022-1711.vid
Proceedings of the First Workshop on Federated Learning for Natural Language Processing (FL4NLP 2022)
Jan 01, 2022
Proceedings of the First Workshop on Federated Learning for Natural Language Processing (FL4NLP 2022). (2022). https://doi.org/10.18653/v1/2022.fl4nlp-1
Optimization of Federated Learning Communications with Heterogeneous Quantization
2022 IEEE 22nd International Conference on Communication Technology (ICCT) / Nov 11, 2022
Liu, P., Gao, T., & Li, C. (2022, November 11). Optimization of Federated Learning Communications with Heterogeneous Quantization. 2022 IEEE 22nd International Conference on Communication Technology (ICCT). https://doi.org/10.1109/icct56141.2022.10073223
Optimization for Data-Driven Learning and Control
Proceedings of the IEEE / Nov 01, 2020
Khan, U. A., Bajwa, W. U., Nedic, A., Rabbat, M. G., & Sayed, A. H. (2020). Optimization for Data-Driven Learning and Control. Proceedings of the IEEE, 108(11), 1863–1868. https://doi.org/10.1109/jproc.2020.3031225
Distributed recursive testing of composite hypothesis in multi-agent networks
Data Fusion in Wireless Sensor Networks: A statistical signal processing perspective / Mar 11, 2019
Distributed recursive testing of composite hypothesis in multi-agent networks. (2019). In Data Fusion in Wireless Sensor Networks: A statistical signal processing perspective (pp. 175–200). Institution of Engineering and Technology. https://doi.org/10.1049/pbce117e_ch8
Carnegie Mellon University
The Grants Register 2018 / Jan 01, 2018
Carnegie Mellon University. (2018). In The Grants Register 2018 (pp. 231–231). Palgrave Macmillan UK. https://doi.org/10.1007/978-1-349-94186-5_299
Queue-based broadcast gossip algorithm for consensus
2016 54th Annual Allerton Conference on Communication, Control, and Computing (Allerton) / Sep 01, 2016
Kar, S., Negi, R., Mahzoon, M., & Sahu, A. K. (2016, September). Queue-based broadcast gossip algorithm for consensus. 2016 54th Annual Allerton Conference on Communication, Control, and Computing (Allerton). https://doi.org/10.1109/allerton.2016.7852379
Attack Resilient Distributed Estimation: A Consensus+Innovations Approach
2018 Annual American Control Conference (ACC) / Jun 01, 2018
Chen, Y., Kar, S., & Moura, J. M. F. (2018, June). Attack Resilient Distributed Estimation: A Consensus+Innovations Approach. 2018 Annual American Control Conference (ACC). https://doi.org/10.23919/acc.2018.8430980
Guest Editorial Inference and Learning over Networks
IEEE Transactions on Signal and Information Processing over Networks / Dec 01, 2016
Matta, V., Richard, C., Saligrama, V., & Sayed, A. H. (2016). Guest Editorial Inference and Learning over Networks. IEEE Transactions on Signal and Information Processing over Networks, 2(4), 423–425. https://doi.org/10.1109/tsipn.2016.2615526
Distributed Sequential Detection for Gaussian Shift-in-Mean Hypothesis Testing
IEEE Transactions on Signal Processing / Jan 01, 2016
Sahu, A. K., & Kar, S. (2016). Distributed Sequential Detection for Gaussian Shift-in-Mean Hypothesis Testing. IEEE Transactions on Signal Processing, 64(1), 89–103. https://doi.org/10.1109/tsp.2015.2478737
Distributed sequential detection for Gaussian binary hypothesis testing: Heterogeneous networks
2014 48th Asilomar Conference on Signals, Systems and Computers / Nov 01, 2014
Sahu, A. K., & Kar, S. (2014, November). Distributed sequential detection for Gaussian binary hypothesis testing: Heterogeneous networks. 2014 48th Asilomar Conference on Signals, Systems and Computers. https://doi.org/10.1109/acssc.2014.7094543
Education
Carnegie Mellon University
PhD, Electrical and Computer Engineering / December, 2018
Experience
Amazon Services LLC
Senior Applied Scientist / October, 2020 — August, 2024
Bosch Center for Artificial Intelligence
Machine Learning Research Scientist / January, 2019 — October, 2020
GE Healthcare AI
Principal AI Scientist / August, 2024 — Present
Join Anit on NotedSource!
Join Now
At NotedSource, we believe that professors, post-docs, scientists and other researchers have deep, untapped knowledge and expertise that can be leveraged to drive innovation within companies. NotedSource is committed to bridging the gap between academia and industry by providing a platform for collaboration with industry and networking with other researchers.
For industry, NotedSource identifies the right academic experts in 24 hours to help organizations build and grow. With a platform of thousands of knowledgeable PhDs, scientists, and industry experts, NotedSource makes connecting and collaborating easy.
For academic researchers such as professors, post-docs, and Ph.D.s, NotedSource provides tools to discover and connect to your colleagues with messaging and news feeds, in addition to the opportunity to be paid for your collaboration with vetted partners.
Expert Institutions
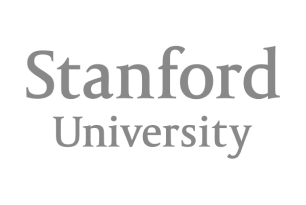
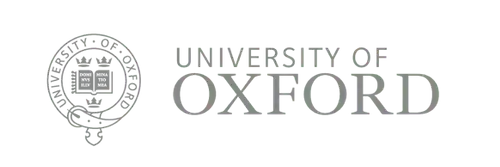
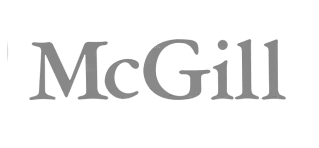
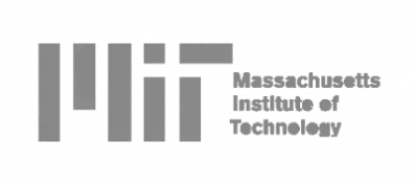
Proudly trusted by
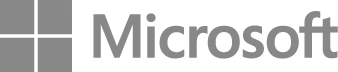

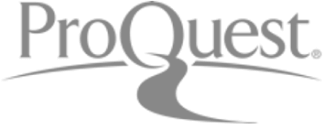
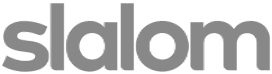

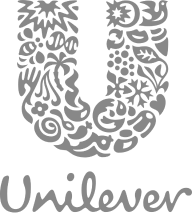