Dhritiman Das, Ph.D.
Postdoctoral Researcher at Massachusetts Institute of Technology : AI | Computer Vision | Signal Processing | Healthcare
Research Expertise
About
Publications
Convolutional Neural Networks for Direct Inference of Pharmacokinetic Parameters: Application to Stroke Dynamic Contrast-Enhanced MRI
Frontiers in Neurology / Jan 08, 2019
Ulas, C., Das, D., Thrippleton, M. J., Valdés Hernández, M. del C., Armitage, P. A., Makin, S. D., Wardlaw, J. M., & Menze, B. H. (2019). Convolutional Neural Networks for Direct Inference of Pharmacokinetic Parameters: Application to Stroke Dynamic Contrast-Enhanced MRI. Frontiers in Neurology, 9. https://doi.org/10.3389/fneur.2018.01147
Quantification of Metabolites in Magnetic Resonance Spectroscopic Imaging Using Machine Learning
Medical Image Computing and Computer Assisted Intervention − MICCAI 2017 / Jan 01, 2017
Das, D., Coello, E., Schulte, R. F., & Menze, B. H. (2017). Quantification of Metabolites in Magnetic Resonance Spectroscopic Imaging Using Machine Learning. In Lecture Notes in Computer Science (pp. 462–470). Springer International Publishing. https://doi.org/10.1007/978-3-319-66179-7_53
Velocity-To-Pressure (V2P) - Net: Inferring Relative Pressures from Time-Varying 3D Fluid Flow Velocities
Lecture Notes in Computer Science / Jan 01, 2021
Shit, S., Das, D., Ezhov, I., Paetzold, J. C., Sanches, A. F., Thuerey, N., & Menze, B. H. (2021). Velocity-To-Pressure (V2P) - Net: Inferring Relative Pressures from Time-Varying 3D Fluid Flow Velocities. In Information Processing in Medical Imaging (pp. 545–558). Springer International Publishing. https://doi.org/10.1007/978-3-030-78191-0_42
An Optical Flow-Based Approach for Minimally Divergent Velocimetry Data Interpolation
International Journal of Biomedical Imaging / Feb 03, 2019
Kanberoglu, B., Das, D., Nair, P., Turaga, P., & Frakes, D. (2019). An Optical Flow-Based Approach for Minimally Divergent Velocimetry Data Interpolation. International Journal of Biomedical Imaging, 2019, 1–14. https://doi.org/10.1155/2019/9435163
Spatially Adaptive Spectral Denoising for MR Spectroscopic Imaging using Frequency-Phase Non-local Means
Lecture Notes in Computer Science / Jan 01, 2016
Das, D., Coello, E., Schulte, R. F., & Menze, B. H. (2016). Spatially Adaptive Spectral Denoising for MR Spectroscopic Imaging using Frequency-Phase Non-local Means. In Medical Image Computing and Computer-Assisted Intervention - MICCAI 2016 (pp. 596–604). Springer International Publishing. https://doi.org/10.1007/978-3-319-46726-9_69
Joint Automatic Metabolite Identification and Quantification of a Set of 1H NMR Spectra
Joint Automatic Metabolite Identification and Quantification of a Set of 1H NMR Spectra. (n.d.). American Chemical Society (ACS). https://doi.org/10.1021/acs.analchem.0c04232.s001
Reliability of MRSI brain temperature mapping at 1.5 and 3 T
NMR in Biomedicine / Nov 24, 2013
Thrippleton, M. J., Parikh, J., Harris, B. A., Hammer, S. J., Semple, S. I. K., Andrews, P. J. D., Wardlaw, J. M., & Marshall, I. (2013). Reliability of MRSI brain temperature mapping at 1.5 and 3 T. NMR in Biomedicine, 27(2), 183–190. Portico. https://doi.org/10.1002/nbm.3050
The Multimodal Brain Tumor Image Segmentation Benchmark (BRATS)
IEEE Transactions on Medical Imaging / Oct 01, 2015
Menze, B. H., Jakab, A., Bauer, S., Kalpathy-Cramer, J., Farahani, K., Kirby, J., Burren, Y., Porz, N., Slotboom, J., Wiest, R., Lanczi, L., Gerstner, E., Weber, M.-A., Arbel, T., Avants, B. B., Ayache, N., Buendia, P., Collins, D. L., Cordier, N., … Van Leemput, K. (2015). The Multimodal Brain Tumor Image Segmentation Benchmark (BRATS). IEEE Transactions on Medical Imaging, 34(10), 1993–2024. https://doi.org/10.1109/tmi.2014.2377694
Education
Technical University of Munich
Ph.D., Computer Science
Arizona State University
M.S., Bioengineering
Manipal Institute of Technology
B.E., Biomedical Engineering
Experience
Massachusetts Institute of Technology
Postdoctoral Researcher
Technical University of Munich
Scientific Staff / 2015 — 2020
GE Healthcare
Early Stage Researcher / 2015 — 2019
Siemens Limited
Computer Vision Intern / May, 2014 — August, 2014
Join Dhritiman on NotedSource!
Join Now
At NotedSource, we believe that professors, post-docs, scientists and other researchers have deep, untapped knowledge and expertise that can be leveraged to drive innovation within companies. NotedSource is committed to bridging the gap between academia and industry by providing a platform for collaboration with industry and networking with other researchers.
For industry, NotedSource identifies the right academic experts in 24 hours to help organizations build and grow. With a platform of thousands of knowledgeable PhDs, scientists, and industry experts, NotedSource makes connecting and collaborating easy.
For academic researchers such as professors, post-docs, and Ph.D.s, NotedSource provides tools to discover and connect to your colleagues with messaging and news feeds, in addition to the opportunity to be paid for your collaboration with vetted partners.
Expert Institutions
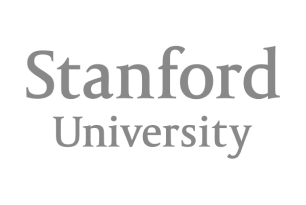
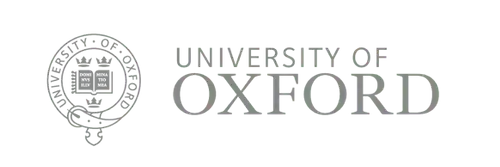
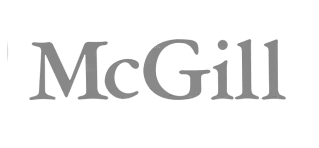
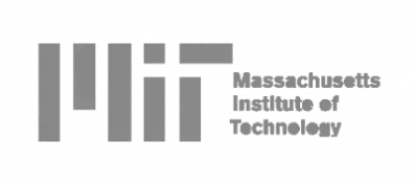
Proudly trusted by
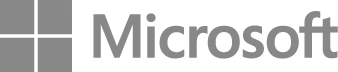

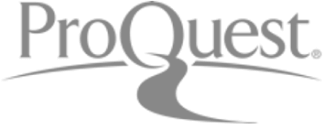
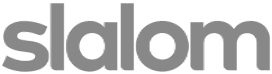

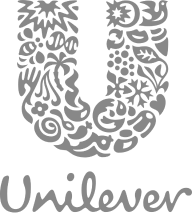