Ping Luo
Bioinformatics Specialist at Princess Margaret Cancer Centre with experience in deep learning
Research Expertise
About
Publications
deepDriver: Predicting Cancer Driver Genes Based on Somatic Mutations Using Deep Convolutional Neural Networks
Frontiers in Genetics / Jan 29, 2019
Luo, P., Ding, Y., Lei, X., & Wu, F.-X. (2019). deepDriver: Predicting Cancer Driver Genes Based on Somatic Mutations Using Deep Convolutional Neural Networks. Frontiers in Genetics, 10. https://doi.org/10.3389/fgene.2019.00013
Enhancing the prediction of disease–gene associations with multimodal deep learning
Bioinformatics / Mar 02, 2019
Luo, P., Li, Y., Tian, L.-P., & Wu, F.-X. (2019). Enhancing the prediction of disease–gene associations with multimodal deep learning. Bioinformatics, 35(19), 3735–3742. https://doi.org/10.1093/bioinformatics/btz155
Disease Gene Prediction by Integrating PPI Networks, Clinical RNA-Seq Data and OMIM Data
IEEE/ACM Transactions on Computational Biology and Bioinformatics / Jan 01, 2019
Luo, P., Tian, L.-P., Ruan, J., & Wu, F.-X. (2019). Disease Gene Prediction by Integrating PPI Networks, Clinical RNA-Seq Data and OMIM Data. IEEE/ACM Transactions on Computational Biology and Bioinformatics, 16(1), 222–232. https://doi.org/10.1109/tcbb.2017.2770120
Identifying Disease-Gene Associations With Graph-Regularized Manifold Learning
Frontiers in Genetics / Apr 02, 2019
Luo, P., Xiao, Q., Wei, P.-J., Liao, B., & Wu, F.-X. (2019). Identifying Disease-Gene Associations With Graph-Regularized Manifold Learning. Frontiers in Genetics, 10. https://doi.org/10.3389/fgene.2019.00270
CReSCENT: CanceR Single Cell ExpressioN Toolkit
Nucleic Acids Research / Jun 01, 2020
Mohanraj, S., Díaz-Mejía, J. J., Pham, M. D., Elrick, H., Husić, M., Rashid, S., Luo, P., Bal, P., Lu, K., Patel, S., Mahalanabis, A., Naidas, A., Christensen, E., Croucher, D., Richards, L. M., Shooshtari, P., Brudno, M., Ramani, A. K., & Pugh, T. J. (2020). CReSCENT: CanceR Single Cell ExpressioN Toolkit. Nucleic Acids Research, 48(W1), W372–W379. https://doi.org/10.1093/nar/gkaa437
CASNMF: A Converged Algorithm for symmetrical nonnegative matrix factorization
Neurocomputing / Jan 01, 2018
Tian, L.-P., Luo, P., Wang, H., Zheng, H., & Wu, F.-X. (2018). CASNMF: A Converged Algorithm for symmetrical nonnegative matrix factorization. Neurocomputing, 275, 2031–2040. https://doi.org/10.1016/j.neucom.2017.10.039
Identifying disease genes from PPI networks weighted by gene expression under different conditions
2016 IEEE International Conference on Bioinformatics and Biomedicine (BIBM) / Dec 01, 2016
Ping Luo, Li-Ping Tian, Jishou Ruan, & Wu, F.-X. (2016). Identifying disease genes from PPI networks weighted by gene expression under different conditions. 2016 IEEE International Conference on Bioinformatics and Biomedicine (BIBM). https://doi.org/10.1109/bibm.2016.7822699
Identifying cell types from single-cell data based on similarities and dissimilarities between cells
BMC Bioinformatics / May 01, 2021
Li, Y., Luo, P., Lu, Y., & Wu, F.-X. (2021). Identifying cell types from single-cell data based on similarities and dissimilarities between cells. BMC Bioinformatics, 22(S3). https://doi.org/10.1186/s12859-020-03873-z
Predicting Gene-Disease Associations with Manifold Learning
Bioinformatics Research and Applications / Jan 01, 2018
Luo, P., Tian, L.-P., Chen, B., Xiao, Q., & Wu, F.-X. (2018). Predicting Gene-Disease Associations with Manifold Learning. Lecture Notes in Computer Science, 265–271. https://doi.org/10.1007/978-3-319-94968-0_26
Ensemble disease gene prediction by clinical sample-based networks
BMC Bioinformatics / Mar 01, 2020
Luo, P., Tian, L.-P., Chen, B., Xiao, Q., & Wu, F.-X. (2020). Ensemble disease gene prediction by clinical sample-based networks. BMC Bioinformatics, 21(S2). https://doi.org/10.1186/s12859-020-3346-8
A Novel Core-Attachment-Based Method to Identify Dynamic Protein Complexes Based on Gene Expression Profiles and PPI Networks
PROTEOMICS / Feb 20, 2019
Xiao, Q., Luo, P., Li, M., Wang, J., & Wu, F.-X. (2019). A Novel Core-Attachment-Based Method to Identify Dynamic Protein Complexes Based on Gene Expression Profiles and PPI Networks. PROTEOMICS, 19(5), 1800129. Portico. https://doi.org/10.1002/pmic.201800129
Predicting disease‐associated genes: Computational methods, databases, and evaluations
WIREs Data Mining and Knowledge Discovery / Jul 28, 2020
Luo, P., Chen, B., Liao, B., & Wu, F. (2020). Predicting disease‐associated genes: Computational methods, databases, and evaluations. WIREs Data Mining and Knowledge Discovery, 11(2). Portico. https://doi.org/10.1002/widm.1383
Normalization of the Immune Microenvironment during Lenalidomide Maintenance Is Associated with Sustained MRD Negativity in Patients with Multiple Myeloma
Blood / Nov 05, 2021
Coffey, D. G., Maura, F., Gonzalez-Kozlova, E., Diaz-Mejia3, J., Luo, P., Zhang, Y., Xu, Y., Warren, E. H., Smith, E. L., Cho, H. J., Lesokhin, A., Diamond, B., Kazandjian, D., Pugh, T. J., Green, D. J., Gnjatic, S., & Landgren, O. (2021). Normalization of the Immune Microenvironment during Lenalidomide Maintenance Is Associated with Sustained MRD Negativity in Patients with Multiple Myeloma. Blood, 138(Supplement 1), 329–329. https://doi.org/10.1182/blood-2021-154506
Network Learning for Biomarker Discovery
International Journal of Network Dynamics and Intelligence / Feb 23, 2023
Ding, Y., Fu, M., Luo, P., & Wu, F.-X. (2023). Network Learning for Biomarker Discovery. International Journal of Network Dynamics and Intelligence. https://doi.org/10.53941/ijndi0201004
Predicting Disease Genes from Clinical Single Sample-Based PPI Networks
Bioinformatics and Biomedical Engineering / Jan 01, 2018
Luo, P., Tian, L.-P., Chen, B., Xiao, Q., & Wu, F.-X. (2018). Predicting Disease Genes from Clinical Single Sample-Based PPI Networks. Lecture Notes in Computer Science, 247–258. https://doi.org/10.1007/978-3-319-78723-7_21
Integrated, Longitudinal Analysis of Cell-free DNA in Uveal Melanoma
Cancer Research Communications / Feb 15, 2023
Wong, D., Luo, P., Znassi, N., Arteaga, D. P., Gray, D., Danesh, A., Han, M., Zhao, E. Y., Pedersen, S., Prokopec, S., Sundaravadanam, Y., Torti, D., Marsh, K., Keshavarzi, S., Xu, W., Krema, H., Joshua, A. M., Butler, M. O., & Pugh, T. J. (2023). Integrated, Longitudinal Analysis of Cell-free DNA in Uveal Melanoma. Cancer Research Communications, 3(2), 267–280. https://doi.org/10.1158/2767-9764.crc-22-0456
Evaluation of single-cell RNAseq labelling algorithms using cancer datasets
Briefings in Bioinformatics / Dec 30, 2022
Christensen, E., Luo, P., Turinsky, A., Husić, M., Mahalanabis, A., Naidas, A., Diaz-Mejia, J. J., Brudno, M., Pugh, T., Ramani, A., & Shooshtari, P. (2022). Evaluation of single-cell RNAseq labelling algorithms using cancer datasets. Briefings in Bioinformatics, 24(1). https://doi.org/10.1093/bib/bbac561
Integrated analysis of cell-free DNA for the early detection of cancer in people with Li-Fraumeni Syndrome
Oct 11, 2022
Wong, D., Luo, P., Oldfield, L., Gong, H., Brunga, L., Rabinowicz, R., Subasri, V., Chan, C., Downs, T., Farncombe, K. M., Luu, B., Norman, M., Eagles, J., Pederson, S., Wellum, J., Danesh, A., Prokopec, S., Zhao, E., Znassi, N., … Pugh, T. J. (2022). Integrated analysis of cell-free DNA for the early detection of cancer in people with Li-Fraumeni Syndrome. https://doi.org/10.1101/2022.10.07.22280848
Improved Spectral Clustering Method for Identifying Cell Types from Single-Cell Data
Intelligent Computing Theories and Application / Jan 01, 2019
Li, Y., Luo, P., Lu, Y., & Wu, F.-X. (2019). Improved Spectral Clustering Method for Identifying Cell Types from Single-Cell Data. Lecture Notes in Computer Science, 177–189. https://doi.org/10.1007/978-3-030-26969-2_17
Multiple Germline Events Contribute to Cancer Development in Patients with Li-Fraumeni Syndrome
Cancer Research Communications / May 01, 2023
Subasri, V., Light, N., Kanwar, N., Brzezinski, J., Luo, P., Hansford, J. R., Cairney, E., Portwine, C., Elser, C., Finlay, J. L., Nichols, K. E., Alon, N., Brunga, L., Anson, J., Kohlmann, W., de Andrade, K. C., Khincha, P. P., Savage, S. A., Schiffman, J. D., … Malkin, D. (2023). Multiple Germline Events Contribute to Cancer Development in Patients with Li-Fraumeni Syndrome. Cancer Research Communications, 3(5), 738–754. https://doi.org/10.1158/2767-9764.crc-22-0402
P087: Integrated analysis of cell-free DNA for the detection of malignant peripheral nerve sheath tumors in patients with neurofibromatosis type 1
Genetics in Medicine Open / Jan 01, 2023
Wong, D., Luo, P., Pederson, S., Chan, C., Farncombe, K., Norman, M., Oldfield, L. E., Kim, R., & Pugh, T. (2023). P087: Integrated analysis of cell-free DNA for the detection of malignant peripheral nerve sheath tumors in patients with neurofibromatosis type 1. Genetics in Medicine Open, 1(1), 100106. https://doi.org/10.1016/j.gimo.2023.100106
OP015: Multi-omic analysis of circulating tumour DNA for the early detection of cancer in patients with Li-Fraumeni syndrome
Genetics in Medicine / Mar 01, 2022
Wong, D., Znassi, N., Luo, P., Oldfield, L. E., Bruce, J., Danesh, A., Prokopec, S., Basra, P., Pederson, S., Wellum, J., Chan, C., Farncombe, K., Norman, M., Brunga, L., Light, N., Shien, A., Subasri, V., Malkin, D., Kim, R., & Pugh, T. (2022). OP015: Multi-omic analysis of circulating tumour DNA for the early detection of cancer in patients with Li-Fraumeni syndrome. Genetics in Medicine, 24(3), S346–S347. https://doi.org/10.1016/j.gim.2022.01.609
Evaluation of single-cell RNA-seq clustering algorithms on cancer tumor datasets
Computational and Structural Biotechnology Journal / Jan 01, 2022
Mahalanabis, A., Turinsky, A. L., Husić, M., Christensen, E., Luo, P., Naidas, A., Brudno, M., Pugh, T., Ramani, A. K., & Shooshtari, P. (2022). Evaluation of single-cell RNA-seq clustering algorithms on cancer tumor datasets. Computational and Structural Biotechnology Journal, 20, 6375–6387. https://doi.org/10.1016/j.csbj.2022.10.029
Education
University of Saskatchewan
Ph.D., Biomedical Engineering / September, 2019
Beijing Institute of Technology
M.Eng., Biomedical Engineering / June, 2015
Hunan University
B.Eng., Computer Science / June, 2010
Experience
Princess Margaret Cancer Centre
Postdoctoral Researcher / November, 2019 — Present
I work in Dr. Trevor Pugh's lab and design cancer diagnosis and treatment strategies by analyze cell-free DNA and single cell sequencing data
Princess Margaret Cancer Centre
Bioinformatics Specialist / September, 2023 — Present
I work in Dr. Tak Mak's lab and study tumor immunology using single cell and TCR sequencing data.
Links & Social Media
Join Ping on NotedSource!
Join Now
At NotedSource, we believe that professors, post-docs, scientists and other researchers have deep, untapped knowledge and expertise that can be leveraged to drive innovation within companies. NotedSource is committed to bridging the gap between academia and industry by providing a platform for collaboration with industry and networking with other researchers.
For industry, NotedSource identifies the right academic experts in 24 hours to help organizations build and grow. With a platform of thousands of knowledgeable PhDs, scientists, and industry experts, NotedSource makes connecting and collaborating easy.
For academic researchers such as professors, post-docs, and Ph.D.s, NotedSource provides tools to discover and connect to your colleagues with messaging and news feeds, in addition to the opportunity to be paid for your collaboration with vetted partners.
Expert Institutions
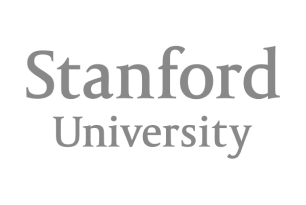
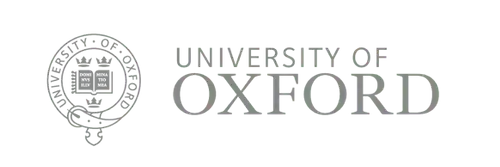
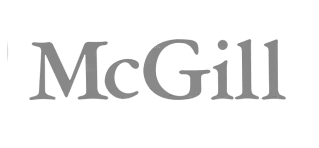
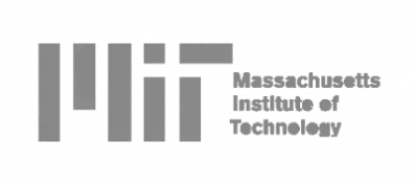
Proudly trusted by
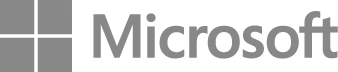

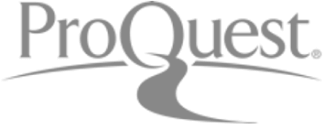
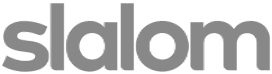

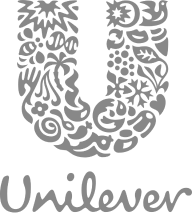