Suhang Wang
Professor at Pennsylvania State University
Research Expertise
About
Publications
Fake News Detection on Social Media
ACM SIGKDD Explorations Newsletter / Sep 01, 2017
Shu, K., Sliva, A., Wang, S., Tang, J., & Liu, H. (2017). Fake News Detection on Social Media. ACM SIGKDD Explorations Newsletter, 19(1), 22–36. https://doi.org/10.1145/3137597.3137600
Feature Selection
ACM Computing Surveys / Dec 06, 2017
Li, J., Cheng, K., Wang, S., Morstatter, F., Trevino, R. P., Tang, J., & Liu, H. (2017). Feature Selection. ACM Computing Surveys, 50(6), 1–45. https://doi.org/10.1145/3136625
FakeNewsNet: A Data Repository with News Content, Social Context, and Spatiotemporal Information for Studying Fake News on Social Media
Big Data / Jun 01, 2020
Shu, K., Mahudeswaran, D., Wang, S., Lee, D., & Liu, H. (2020). FakeNewsNet: A Data Repository with News Content, Social Context, and Spatiotemporal Information for Studying Fake News on Social Media. Big Data, 8(3), 171–188. https://doi.org/10.1089/big.2020.0062
Beyond News Contents
Proceedings of the Twelfth ACM International Conference on Web Search and Data Mining / Jan 30, 2019
Shu, K., Wang, S., & Liu, H. (2019). Beyond News Contents. Proceedings of the Twelfth ACM International Conference on Web Search and Data Mining. https://doi.org/10.1145/3289600.3290994
dEFEND
Proceedings of the 25th ACM SIGKDD International Conference on Knowledge Discovery & Data Mining / Jul 25, 2019
Shu, K., Cui, L., Wang, S., Lee, D., & Liu, H. (2019). dEFEND. Proceedings of the 25th ACM SIGKDD International Conference on Knowledge Discovery & Data Mining. https://doi.org/10.1145/3292500.3330935
Understanding User Profiles on Social Media for Fake News Detection
2018 IEEE Conference on Multimedia Information Processing and Retrieval (MIPR) / Apr 01, 2018
Shu, K., Wang, S., & Liu, H. (2018). Understanding User Profiles on Social Media for Fake News Detection. 2018 IEEE Conference on Multimedia Information Processing and Retrieval (MIPR). https://doi.org/10.1109/mipr.2018.00092
Graph Structure Learning for Robust Graph Neural Networks
Proceedings of the 26th ACM SIGKDD International Conference on Knowledge Discovery & Data Mining / Aug 20, 2020
Jin, W., Ma, Y., Liu, X., Tang, X., Wang, S., & Tang, J. (2020). Graph Structure Learning for Robust Graph Neural Networks. Proceedings of the 26th ACM SIGKDD International Conference on Knowledge Discovery & Data Mining. https://doi.org/10.1145/3394486.3403049
Unsupervised Fake News Detection on Social Media: A Generative Approach
Proceedings of the AAAI Conference on Artificial Intelligence / Jul 17, 2019
Yang, S., Shu, K., Wang, S., Gu, R., Wu, F., & Liu, H. (2019). Unsupervised Fake News Detection on Social Media: A Generative Approach. Proceedings of the AAAI Conference on Artificial Intelligence, 33(01), 5644–5651. https://doi.org/10.1609/aaai.v33i01.33015644
What Your Images Reveal
Proceedings of the 26th International Conference on World Wide Web / Apr 03, 2017
Wang, S., Wang, Y., Tang, J., Shu, K., Ranganath, S., & Liu, H. (2017). What Your Images Reveal. Proceedings of the 26th International Conference on World Wide Web. https://doi.org/10.1145/3038912.3052638
Signed Network Embedding in Social Media
Proceedings of the 2017 SIAM International Conference on Data Mining / Jun 09, 2017
Wang, S., Tang, J., Aggarwal, C., Chang, Y., & Liu, H. (2017). Signed Network Embedding in Social Media. Proceedings of the 2017 SIAM International Conference on Data Mining, 327–335. https://doi.org/10.1137/1.9781611974973.37
User Identity Linkage across Online Social Networks
ACM SIGKDD Explorations Newsletter / Mar 22, 2017
Shu, K., Wang, S., Tang, J., Zafarani, R., & Liu, H. (2017). User Identity Linkage across Online Social Networks. ACM SIGKDD Explorations Newsletter, 18(2), 5–17. https://doi.org/10.1145/3068777.3068781
Embedded Unsupervised Feature Selection
Proceedings of the AAAI Conference on Artificial Intelligence / Feb 10, 2015
Wang, S., Tang, J., & Liu, H. (2015). Embedded Unsupervised Feature Selection. Proceedings of the AAAI Conference on Artificial Intelligence, 29(1). https://doi.org/10.1609/aaai.v29i1.9211
Preprint repository arXiv achieves milestone million uploads
Physics Today / Jan 01, 2014
Preprint repository arXiv achieves milestone million uploads. (2014). Physics Today. https://doi.org/10.1063/pt.5.028530
The role of user profiles for fake news detection
Proceedings of the 2019 IEEE/ACM International Conference on Advances in Social Networks Analysis and Mining / Aug 27, 2019
Shu, K., Zhou, X., Wang, S., Zafarani, R., & Liu, H. (2019). The role of user profiles for fake news detection. Proceedings of the 2019 IEEE/ACM International Conference on Advances in Social Networks Analysis and Mining. https://doi.org/10.1145/3341161.3342927
Sentiment Analysis for Social Media Images
2015 IEEE International Conference on Data Mining Workshop (ICDMW) / Nov 01, 2015
Wang, Y., & Li, B. (2015). Sentiment Analysis for Social Media Images. 2015 IEEE International Conference on Data Mining Workshop (ICDMW). https://doi.org/10.1109/icdmw.2015.142
Learning Word Representations for Sentiment Analysis
Cognitive Computation / Aug 17, 2017
Li, Y., Pan, Q., Yang, T., Wang, S., Tang, J., & Cambria, E. (2017). Learning Word Representations for Sentiment Analysis. Cognitive Computation, 9(6), 843–851. https://doi.org/10.1007/s12559-017-9492-2
Hierarchical Propagation Networks for Fake News Detection: Investigation and Exploitation
Proceedings of the International AAAI Conference on Web and Social Media / May 26, 2020
Shu, K., Mahudeswaran, D., Wang, S., & Liu, H. (2020). Hierarchical Propagation Networks for Fake News Detection: Investigation and Exploitation. Proceedings of the International AAAI Conference on Web and Social Media, 14, 626–637. https://doi.org/10.1609/icwsm.v14i1.7329
Adversarial Attacks on Graph Neural Networks via Node Injections: A Hierarchical Reinforcement Learning Approach
Proceedings of The Web Conference 2020 / Apr 20, 2020
Sun, Y., Wang, S., Tang, X., Hsieh, T.-Y., & Honavar, V. (2020). Adversarial Attacks on Graph Neural Networks via Node Injections: A Hierarchical Reinforcement Learning Approach. Proceedings of The Web Conference 2020. https://doi.org/10.1145/3366423.3380149
A Generative Model for category text generation
Information Sciences / Jun 01, 2018
Li, Y., Pan, Q., Wang, S., Yang, T., & Cambria, E. (2018). A Generative Model for category text generation. Information Sciences, 450, 301–315. https://doi.org/10.1016/j.ins.2018.03.050
GraphSMOTE: Imbalanced Node Classification on Graphs with Graph Neural Networks
Proceedings of the 14th ACM International Conference on Web Search and Data Mining / Mar 08, 2021
Zhao, T., Zhang, X., & Wang, S. (2021). GraphSMOTE: Imbalanced Node Classification on Graphs with Graph Neural Networks. Proceedings of the 14th ACM International Conference on Web Search and Data Mining. https://doi.org/10.1145/3437963.3441720
About the social role of child and adolescent psychiatrists in times of epidemic
IACAPAP ArXiv / Jan 01, 2020
Falissard, B. (2020). About the social role of child and adolescent psychiatrists in times of epidemic. IACAPAP ArXiv. https://doi.org/10.14744/iacapaparxiv.2020.20004
Transferring Robustness for Graph Neural Network Against Poisoning Attacks
Proceedings of the 13th International Conference on Web Search and Data Mining / Jan 20, 2020
Tang, X., Li, Y., Sun, Y., Yao, H., Mitra, P., & Wang, S. (2020). Transferring Robustness for Graph Neural Network Against Poisoning Attacks. Proceedings of the 13th International Conference on Web Search and Data Mining. https://doi.org/10.1145/3336191.3371851
Say No to the Discrimination: Learning Fair Graph Neural Networks with Limited Sensitive Attribute Information
Proceedings of the 14th ACM International Conference on Web Search and Data Mining / Mar 08, 2021
Dai, E., & Wang, S. (2021). Say No to the Discrimination: Learning Fair Graph Neural Networks with Limited Sensitive Attribute Information. Proceedings of the 14th ACM International Conference on Web Search and Data Mining. https://doi.org/10.1145/3437963.3441752
Discriminative graph regularized extreme learning machine and its application to face recognition
Neurocomputing / Feb 01, 2015
Peng, Y., Wang, S., Long, X., & Lu, B.-L. (2015). Discriminative graph regularized extreme learning machine and its application to face recognition. Neurocomputing, 149, 340–353. https://doi.org/10.1016/j.neucom.2013.12.065
Linked Document Embedding for Classification
Proceedings of the 25th ACM International on Conference on Information and Knowledge Management / Oct 24, 2016
Wang, S., Tang, J., Aggarwal, C., & Liu, H. (2016). Linked Document Embedding for Classification. Proceedings of the 25th ACM International on Conference on Information and Knowledge Management. https://doi.org/10.1145/2983323.2983755
Recommendation with Social Dimensions
Proceedings of the AAAI Conference on Artificial Intelligence / Feb 21, 2016
Tang, J., Wang, S., Hu, X., Yin, D., Bi, Y., Chang, Y., & Liu, H. (2016). Recommendation with Social Dimensions. Proceedings of the AAAI Conference on Artificial Intelligence, 30(1). https://doi.org/10.1609/aaai.v30i1.9976
Graph Few-Shot Learning via Knowledge Transfer
Proceedings of the AAAI Conference on Artificial Intelligence / Apr 03, 2020
Yao, H., Zhang, C., Wei, Y., Jiang, M., Wang, S., Huang, J., Chawla, N., & Li, Z. (2020). Graph Few-Shot Learning via Knowledge Transfer. Proceedings of the AAAI Conference on Artificial Intelligence, 34(04), 6656–6663. https://doi.org/10.1609/aaai.v34i04.6142
SAME
Proceedings of the 2019 IEEE/ACM International Conference on Advances in Social Networks Analysis and Mining / Aug 27, 2019
Cui, L., Wang, S., & Lee, D. (2019). SAME. Proceedings of the 2019 IEEE/ACM International Conference on Advances in Social Networks Analysis and Mining. https://doi.org/10.1145/3341161.3342894
Attributed Signed Network Embedding
Proceedings of the 2017 ACM on Conference on Information and Knowledge Management / Nov 06, 2017
Wang, S., Aggarwal, C., Tang, J., & Liu, H. (2017). Attributed Signed Network Embedding. Proceedings of the 2017 ACM on Conference on Information and Knowledge Management. https://doi.org/10.1145/3132847.3132905
DETERRENT: Knowledge Guided Graph Attention Network for Detecting Healthcare Misinformation
Proceedings of the 26th ACM SIGKDD International Conference on Knowledge Discovery & Data Mining / Aug 20, 2020
Cui, L., Seo, H., Tabar, M., Ma, F., Wang, S., & Lee, D. (2020). DETERRENT: Knowledge Guided Graph Attention Network for Detecting Healthcare Misinformation. Proceedings of the 26th ACM SIGKDD International Conference on Knowledge Discovery & Data Mining. https://doi.org/10.1145/3394486.3403092
Joint Modeling of Local and Global Temporal Dynamics for Multivariate Time Series Forecasting with Missing Values
Proceedings of the AAAI Conference on Artificial Intelligence / Apr 03, 2020
Tang, X., Yao, H., Sun, Y., Aggarwal, C., Mitra, P., & Wang, S. (2020). Joint Modeling of Local and Global Temporal Dynamics for Multivariate Time Series Forecasting with Missing Values. Proceedings of the AAAI Conference on Artificial Intelligence, 34(04), 5956–5963. https://doi.org/10.1609/aaai.v34i04.6056
ArXiv preprint server plans multimillion-dollar overhaul
Nature / Jun 29, 2016
Van Noorden, R. (2016). ArXiv preprint server plans multimillion-dollar overhaul. Nature, 534(7609), 602–602. https://doi.org/10.1038/534602a
Mining Disinformation and Fake News: Concepts, Methods, and Recent Advancements
Lecture Notes in Social Networks / Jan 01, 2020
Shu, K., Wang, S., Lee, D., & Liu, H. (2020). Mining Disinformation and Fake News: Concepts, Methods, and Recent Advancements. Disinformation, Misinformation, and Fake News in Social Media, 1–19. https://doi.org/10.1007/978-3-030-42699-6_1
Ginger Cannot Cure Cancer: Battling Fake Health News with a Comprehensive Data Repository
Proceedings of the International AAAI Conference on Web and Social Media / May 26, 2020
Dai, E., Sun, Y., & Wang, S. (2020). Ginger Cannot Cure Cancer: Battling Fake Health News with a Comprehensive Data Repository. Proceedings of the International AAAI Conference on Web and Social Media, 14, 853–862. https://doi.org/10.1609/icwsm.v14i1.7350
Learning binary codes with neural collaborative filtering for efficient recommendation systems
Knowledge-Based Systems / May 01, 2019
Li, Y., Wang, S., Pan, Q., Peng, H., Yang, T., & Cambria, E. (2019). Learning binary codes with neural collaborative filtering for efficient recommendation systems. Knowledge-Based Systems, 172, 64–75. https://doi.org/10.1016/j.knosys.2019.02.012
Personalized Privacy-Preserving Social Recommendation
Proceedings of the AAAI Conference on Artificial Intelligence / Apr 29, 2018
Meng, X., Wang, S., Shu, K., Li, J., Chen, B., Liu, H., & Zhang, Y. (2018). Personalized Privacy-Preserving Social Recommendation. Proceedings of the AAAI Conference on Artificial Intelligence, 32(1). https://doi.org/10.1609/aaai.v32i1.11714
Toward Dual Roles of Users in Recommender Systems
Proceedings of the 24th ACM International on Conference on Information and Knowledge Management / Oct 17, 2015
Wang, S., Tang, J., & Liu, H. (2015). Toward Dual Roles of Users in Recommender Systems. Proceedings of the 24th ACM International on Conference on Information and Knowledge Management. https://doi.org/10.1145/2806416.2806520
Disentangled Variational Auto-Encoder for semi-supervised learning
Information Sciences / May 01, 2019
Li, Y., Pan, Q., Wang, S., Peng, H., Yang, T., & Cambria, E. (2019). Disentangled Variational Auto-Encoder for semi-supervised learning. Information Sciences, 482, 73–85. https://doi.org/10.1016/j.ins.2018.12.057
Graph Adversarial Attack via Rewiring
Proceedings of the 27th ACM SIGKDD Conference on Knowledge Discovery & Data Mining / Aug 14, 2021
Ma, Y., Wang, S., Derr, T., Wu, L., & Tang, J. (2021). Graph Adversarial Attack via Rewiring. Proceedings of the 27th ACM SIGKDD Conference on Knowledge Discovery & Data Mining. https://doi.org/10.1145/3447548.3467416
Exploring Hierarchical Structures for Recommender Systems
IEEE Transactions on Knowledge and Data Engineering / Jun 01, 2018
Wang, S., Tang, J., Wang, Y., & Liu, H. (2018). Exploring Hierarchical Structures for Recommender Systems. IEEE Transactions on Knowledge and Data Engineering, 30(6), 1022–1035. https://doi.org/10.1109/tkde.2018.2789443
Deep Headline Generation for Clickbait Detection
2018 IEEE International Conference on Data Mining (ICDM) / Nov 01, 2018
Shu, K., Wang, S., Le, T., Lee, D., & Liu, H. (2018). Deep Headline Generation for Clickbait Detection. 2018 IEEE International Conference on Data Mining (ICDM). https://doi.org/10.1109/icdm.2018.00062
Exploiting Emotional Information for Trust/Distrust Prediction
Proceedings of the 2016 SIAM International Conference on Data Mining / Jun 30, 2016
Beigi, G., Tang, J., Wang, S., & Liu, H. (2016). Exploiting Emotional Information for Trust/Distrust Prediction. Proceedings of the 2016 SIAM International Conference on Data Mining. https://doi.org/10.1137/1.9781611974348.10
MALCOM: Generating Malicious Comments to Attack Neural Fake News Detection Models
2020 IEEE International Conference on Data Mining (ICDM) / Nov 01, 2020
Le, T., Wang, S., & Lee, D. (2020). MALCOM: Generating Malicious Comments to Attack Neural Fake News Detection Models. 2020 IEEE International Conference on Data Mining (ICDM). https://doi.org/10.1109/icdm50108.2020.00037
Enhanced low-rank representation via sparse manifold adaption for semi-supervised learning
Neural Networks / May 01, 2015
Peng, Y., Lu, B.-L., & Wang, S. (2015). Enhanced low-rank representation via sparse manifold adaption for semi-supervised learning. Neural Networks, 65, 1–17. https://doi.org/10.1016/j.neunet.2015.01.001
MEGAN: A Generative Adversarial Network for Multi-View Network Embedding
Proceedings of the Twenty-Eighth International Joint Conference on Artificial Intelligence / Aug 01, 2019
Sun, Y., Wang, S., Hsieh, T.-Y., Tang, X., & Honavar, V. (2019). MEGAN: A Generative Adversarial Network for Multi-View Network Embedding. Proceedings of the Twenty-Eighth International Joint Conference on Artificial Intelligence. https://doi.org/10.24963/ijcai.2019/489
Using a Random Forest to Inspire a Neural Network and Improving on It
Proceedings of the 2017 SIAM International Conference on Data Mining / Jun 09, 2017
Wang, S., Aggarwal, C., & Liu, H. (2017). Using a Random Forest to Inspire a Neural Network and Improving on It. Proceedings of the 2017 SIAM International Conference on Data Mining, 1–9. https://doi.org/10.1137/1.9781611974973.1
Feature Selection
Encyclopedia of Machine Learning and Data Mining / Jan 01, 2016
Wang, S., Tang, J., & Liu, H. (2016). Feature Selection. Encyclopedia of Machine Learning and Data Mining, 1–9. https://doi.org/10.1007/978-1-4899-7502-7_101-1
GRACE: Generating Concise and Informative Contrastive Sample to Explain Neural Network Model's Prediction
Proceedings of the 26th ACM SIGKDD International Conference on Knowledge Discovery & Data Mining / Aug 20, 2020
Le, T., Wang, S., & Lee, D. (2020). GRACE: Generating Concise and Informative Contrastive Sample to Explain Neural Network Model’s Prediction. Proceedings of the 26th ACM SIGKDD International Conference on Knowledge Discovery & Data Mining. https://doi.org/10.1145/3394486.3403066
Explainable Multivariate Time Series Classification
Proceedings of the 14th ACM International Conference on Web Search and Data Mining / Mar 08, 2021
Hsieh, T.-Y., Wang, S., Sun, Y., & Honavar, V. (2021). Explainable Multivariate Time Series Classification. Proceedings of the 14th ACM International Conference on Web Search and Data Mining. https://doi.org/10.1145/3437963.3441815
CrossFire
Proceedings of the Eleventh ACM International Conference on Web Search and Data Mining / Feb 02, 2018
Shu, K., Wang, S., Tang, J., Wang, Y., & Liu, H. (2018). CrossFire. Proceedings of the Eleventh ACM International Conference on Web Search and Data Mining. https://doi.org/10.1145/3159652.3159692
PPP: Joint Pointwise and Pairwise Image Label Prediction
2016 IEEE Conference on Computer Vision and Pattern Recognition (CVPR) / Jun 01, 2016
Wang, Y., Wang, S., Tang, J., Liu, H., & Li, B. (2016). PPP: Joint Pointwise and Pairwise Image Label Prediction. 2016 IEEE Conference on Computer Vision and Pattern Recognition (CVPR). https://doi.org/10.1109/cvpr.2016.646
dEFEND
Proceedings of the 28th ACM International Conference on Information and Knowledge Management / Nov 03, 2019
Cui, L., Shu, K., Wang, S., Lee, D., & Liu, H. (2019). dEFEND. Proceedings of the 28th ACM International Conference on Information and Knowledge Management. https://doi.org/10.1145/3357384.3357862
NRGNN
Proceedings of the 27th ACM SIGKDD Conference on Knowledge Discovery & Data Mining / Aug 14, 2021
Dai, E., Aggarwal, C., & Wang, S. (2021). NRGNN. Proceedings of the 27th ACM SIGKDD Conference on Knowledge Discovery & Data Mining. https://doi.org/10.1145/3447548.3467364
CLARE: A Joint Approach to Label Classification and Tag Recommendation
Proceedings of the AAAI Conference on Artificial Intelligence / Feb 10, 2017
Wang, Y., Wang, S., Tang, J., Qi, G., Liu, H., & Li, B. (2017). CLARE: A Joint Approach to Label Classification and Tag Recommendation. Proceedings of the AAAI Conference on Artificial Intelligence, 31(1). https://doi.org/10.1609/aaai.v31i1.10479
Learning How to Propagate Messages in Graph Neural Networks
Proceedings of the 27th ACM SIGKDD Conference on Knowledge Discovery & Data Mining / Aug 14, 2021
Xiao, T., Chen, Z., Wang, D., & Wang, S. (2021). Learning How to Propagate Messages in Graph Neural Networks. Proceedings of the 27th ACM SIGKDD Conference on Knowledge Discovery & Data Mining. https://doi.org/10.1145/3447548.3467451
Unsupervised Representation Learning of Spatial Data via Multimodal Embedding
Proceedings of the 28th ACM International Conference on Information and Knowledge Management / Nov 03, 2019
Jenkins, P., Farag, A., Wang, S., & Li, Z. (2019). Unsupervised Representation Learning of Spatial Data via Multimodal Embedding. Proceedings of the 28th ACM International Conference on Information and Knowledge Management. https://doi.org/10.1145/3357384.3358001
Knowing your FATE
Proceedings of the 26th ACM SIGKDD International Conference on Knowledge Discovery & Data Mining / Aug 20, 2020
Tang, X., Liu, Y., Shah, N., Shi, X., Mitra, P., & Wang, S. (2020). Knowing your FATE. Proceedings of the 26th ACM SIGKDD International Conference on Knowledge Discovery & Data Mining. https://doi.org/10.1145/3394486.3403276
Towards Interpretation of Recommender Systems with Sorted Explanation Paths
2018 IEEE International Conference on Data Mining (ICDM) / Nov 01, 2018
Yang, F., Liu, N., Wang, S., & Hu, X. (2018). Towards Interpretation of Recommender Systems with Sorted Explanation Paths. 2018 IEEE International Conference on Data Mining (ICDM). https://doi.org/10.1109/icdm.2018.00082
Fairness, explainability, privacy, and robustness for trustworthy algorithmic decision-making
Big Data Analytics in Chemoinformatics and Bioinformatics / Jan 01, 2023
Majumdar, S. (2023). Fairness, explainability, privacy, and robustness for trustworthy algorithmic decision-making. Big Data Analytics in Chemoinformatics and Bioinformatics, 61–95. https://doi.org/10.1016/b978-0-323-85713-0.00017-7
Towards Self-Explainable Graph Neural Network
Proceedings of the 30th ACM International Conference on Information & Knowledge Management / Oct 26, 2021
Dai, E., & Wang, S. (2021). Towards Self-Explainable Graph Neural Network. Proceedings of the 30th ACM International Conference on Information & Knowledge Management. https://doi.org/10.1145/3459637.3482306
Privacy Preserving Text Representation Learning
Proceedings of the 30th ACM Conference on Hypertext and Social Media / Sep 12, 2019
Beigi, G., Shu, K., Guo, R., Wang, S., & Liu, H. (2019). Privacy Preserving Text Representation Learning. Proceedings of the 30th ACM Conference on Hypertext and Social Media. https://doi.org/10.1145/3342220.3344925
Towards privacy preserving social recommendation under personalized privacy settings
World Wide Web / Jul 14, 2018
Meng, X., Wang, S., Shu, K., Li, J., Chen, B., Liu, H., & Zhang, Y. (2018). Towards privacy preserving social recommendation under personalized privacy settings. World Wide Web, 22(6), 2853–2881. https://doi.org/10.1007/s11280-018-0620-z
Random-Forest-Inspired Neural Networks
ACM Transactions on Intelligent Systems and Technology / Oct 29, 2018
Wang, S., Aggarwal, C., & Liu, H. (2018). Random-Forest-Inspired Neural Networks. ACM Transactions on Intelligent Systems and Technology, 9(6), 1–25. https://doi.org/10.1145/3232230
Paired Restricted Boltzmann Machine for Linked Data
Proceedings of the 25th ACM International on Conference on Information and Knowledge Management / Oct 24, 2016
Wang, S., Tang, J., Morstatter, F., & Liu, H. (2016). Paired Restricted Boltzmann Machine for Linked Data. Proceedings of the 25th ACM International on Conference on Information and Knowledge Management. https://doi.org/10.1145/2983323.2983756
Deep Multi-Graph Clustering via Attentive Cross-Graph Association
Proceedings of the 13th International Conference on Web Search and Data Mining / Jan 20, 2020
Luo, D., Ni, J., Wang, S., Bian, Y., Yu, X., & Zhang, X. (2020). Deep Multi-Graph Clustering via Attentive Cross-Graph Association. Proceedings of the 13th International Conference on Web Search and Data Mining. https://doi.org/10.1145/3336191.3371806
Exploiting Emotion on Reviews for Recommender Systems
Proceedings of the AAAI Conference on Artificial Intelligence / Apr 29, 2018
Meng, X., Wang, S., Liu, H., & Zhang, Y. (2018). Exploiting Emotion on Reviews for Recommender Systems. Proceedings of the AAAI Conference on Artificial Intelligence, 32(1). https://doi.org/10.1609/aaai.v32i1.11685
Linked Variational AutoEncoders for Inferring Substitutable and Supplementary Items
Proceedings of the Twelfth ACM International Conference on Web Search and Data Mining / Jan 30, 2019
Rakesh, V., Wang, S., Shu, K., & Liu, H. (2019). Linked Variational AutoEncoders for Inferring Substitutable and Supplementary Items. Proceedings of the Twelfth ACM International Conference on Web Search and Data Mining. https://doi.org/10.1145/3289600.3290963
Facilitating Time Critical Information Seeking in Social Media
IEEE Transactions on Knowledge and Data Engineering / Oct 01, 2017
Ranganath, S., Wang, S., Hu, X., Tang, J., & Liu, H. (2017). Facilitating Time Critical Information Seeking in Social Media. IEEE Transactions on Knowledge and Data Engineering, 29(10), 2197–2209. https://doi.org/10.1109/tkde.2017.2701375
Predicting Online Protest Participation of Social Media Users
Proceedings of the AAAI Conference on Artificial Intelligence / Feb 21, 2016
Ranganath, S., Morstatter, F., Hu, X., Tang, J., Wang, S., & Liu, H. (2016). Predicting Online Protest Participation of Social Media Users. Proceedings of the AAAI Conference on Artificial Intelligence, 30(1). https://doi.org/10.1609/aaai.v30i1.9988
Times series forecasting for urban building energy consumption based on graph convolutional network
Applied Energy / Feb 01, 2022
Hu, Y., Cheng, X., Wang, S., Chen, J., Zhao, T., & Dai, E. (2022). Times series forecasting for urban building energy consumption based on graph convolutional network. Applied Energy, 307, 118231. https://doi.org/10.1016/j.apenergy.2021.118231
Towards Robust Graph Neural Networks for Noisy Graphs with Sparse Labels
Proceedings of the Fifteenth ACM International Conference on Web Search and Data Mining / Feb 11, 2022
Dai, E., Jin, W., Liu, H., & Wang, S. (2022). Towards Robust Graph Neural Networks for Noisy Graphs with Sparse Labels. Proceedings of the Fifteenth ACM International Conference on Web Search and Data Mining. https://doi.org/10.1145/3488560.3498408
Semi-Supervised Graph-to-Graph Translation
Proceedings of the 29th ACM International Conference on Information & Knowledge Management / Oct 19, 2020
Zhao, T., Tang, X., Zhang, X., & Wang, S. (2020). Semi-Supervised Graph-to-Graph Translation. Proceedings of the 29th ACM International Conference on Information & Knowledge Management. https://doi.org/10.1145/3340531.3411977
Identifying Rhetorical Questions in Social Media
Proceedings of the International AAAI Conference on Web and Social Media / Aug 04, 2021
Ranganath, S., Hu, X., Tang, J., Wang, S., & Liu, H. (2021). Identifying Rhetorical Questions in Social Media. Proceedings of the International AAAI Conference on Web and Social Media, 10(1), 667–670. https://doi.org/10.1609/icwsm.v10i1.14771
Towards Fair Classifiers Without Sensitive Attributes
Proceedings of the Fifteenth ACM International Conference on Web Search and Data Mining / Feb 11, 2022
Zhao, T., Dai, E., Shu, K., & Wang, S. (2022). Towards Fair Classifiers Without Sensitive Attributes. Proceedings of the Fifteenth ACM International Conference on Web Search and Data Mining. https://doi.org/10.1145/3488560.3498493
Opinions Power Opinions: Joint Link and Interaction Polarity Predictions in Signed Networks
2018 IEEE/ACM International Conference on Advances in Social Networks Analysis and Mining (ASONAM) / Aug 01, 2018
Derr, T., Wang, Z., & Tang, J. (2018). Opinions Power Opinions: Joint Link and Interaction Polarity Predictions in Signed Networks. 2018 IEEE/ACM International Conference on Advances in Social Networks Analysis and Mining (ASONAM). https://doi.org/10.1109/asonam.2018.8508263
Popularity prediction on vacation rental websites
Neurocomputing / Oct 01, 2020
Li, Y., Wang, S., Ma, Y., Pan, Q., & Cambria, E. (2020). Popularity prediction on vacation rental websites. Neurocomputing, 412, 372–380. https://doi.org/10.1016/j.neucom.2020.05.092
Understanding and Identifying Rhetorical Questions in Social Media
ACM Transactions on Intelligent Systems and Technology / Jan 10, 2018
Ranganath, S., Hu, X., Tang, J., Wang, S., & Liu, H. (2018). Understanding and Identifying Rhetorical Questions in Social Media. ACM Transactions on Intelligent Systems and Technology, 9(2), 1–22. https://doi.org/10.1145/3108364
Attacking Black-box Recommendations via Copying Cross-domain User Profiles
2021 IEEE 37th International Conference on Data Engineering (ICDE) / Apr 01, 2021
Fan, W., Derr, T., Zhao, X., Ma, Y., Liu, H., Wang, J., Tang, J., & Li, Q. (2021). Attacking Black-box Recommendations via Copying Cross-domain User Profiles. 2021 IEEE 37th International Conference on Data Engineering (ICDE). https://doi.org/10.1109/icde51399.2021.00140
Exploring Edge Disentanglement for Node Classification
Proceedings of the ACM Web Conference 2022 / Apr 25, 2022
Zhao, T., Zhang, X., & Wang, S. (2022). Exploring Edge Disentanglement for Node Classification. Proceedings of the ACM Web Conference 2022. https://doi.org/10.1145/3485447.3511929
Semi-supervised anomaly detection in dynamic communication networks
Information Sciences / Sep 01, 2021
Meng, X., Wang, S., Liang, Z., Yao, D., Zhou, J., & Zhang, Y. (2021). Semi-supervised anomaly detection in dynamic communication networks. Information Sciences, 571, 527–542. https://doi.org/10.1016/j.ins.2021.04.056
Exploiting Hierarchical Structures for Unsupervised Feature Selection
Proceedings of the 2017 SIAM International Conference on Data Mining / Jun 09, 2017
Wang, S., Wang, Y., Tang, J., Aggarwal, C., Ranganath, S., & Liu, H. (2017). Exploiting Hierarchical Structures for Unsupervised Feature Selection. Proceedings of the 2017 SIAM International Conference on Data Mining, 507–515. https://doi.org/10.1137/1.9781611974973.57
Weakly Supervised Facial Attribute Manipulation via Deep Adversarial Network
2018 IEEE Winter Conference on Applications of Computer Vision (WACV) / Mar 01, 2018
Wang, Y., Wang, S., Qi, G., Tang, J., & Li, B. (2018). Weakly Supervised Facial Attribute Manipulation via Deep Adversarial Network. 2018 IEEE Winter Conference on Applications of Computer Vision (WACV). https://doi.org/10.1109/wacv.2018.00019
Towards Unbiased and Robust Causal Ranking for Recommender Systems
Proceedings of the Fifteenth ACM International Conference on Web Search and Data Mining / Feb 11, 2022
Xiao, T., & Wang, S. (2022). Towards Unbiased and Robust Causal Ranking for Recommender Systems. Proceedings of the Fifteenth ACM International Conference on Web Search and Data Mining. https://doi.org/10.1145/3488560.3498521
Randomized Feature Engineering as a Fast and Accurate Alternative to Kernel Methods
Proceedings of the 23rd ACM SIGKDD International Conference on Knowledge Discovery and Data Mining / Aug 04, 2017
Wang, S., Aggarwal, C., & Liu, H. (2017). Randomized Feature Engineering as a Fast and Accurate Alternative to Kernel Methods. Proceedings of the 23rd ACM SIGKDD International Conference on Knowledge Discovery and Data Mining. https://doi.org/10.1145/3097983.3098001
Reconstruction-based Unsupervised Feature Selection: An Embedded Approach
Proceedings of the Twenty-Sixth International Joint Conference on Artificial Intelligence / Aug 01, 2017
Li, J., Tang, J., & Liu, H. (2017). Reconstruction-based Unsupervised Feature Selection: An Embedded Approach. Proceedings of the Twenty-Sixth International Joint Conference on Artificial Intelligence. https://doi.org/10.24963/ijcai.2017/300
Price Recommendation on Vacation Rental Websites
Proceedings of the 2017 SIAM International Conference on Data Mining / Jun 09, 2017
Li, Y., Wang, S., Yang, T., Pan, Q., & Tang, J. (2017). Price Recommendation on Vacation Rental Websites. Proceedings of the 2017 SIAM International Conference on Data Mining, 399–407. https://doi.org/10.1137/1.9781611974973.45
Self-Supervised learning for Conversational Recommendation
Information Processing & Management / Nov 01, 2022
Li, S., Xie, R., Zhu, Y., Zhuang, F., Tang, Z., Zhao, W. X., & He, Q. (2022). Self-Supervised learning for Conversational Recommendation. Information Processing & Management, 59(6), 103067. https://doi.org/10.1016/j.ipm.2022.103067
HP-GMN: Graph Memory Networks for Heterophilous Graphs
2022 IEEE International Conference on Data Mining (ICDM) / Nov 01, 2022
Xu, J., Dai, E., Zhang, X., & Wang, S. (2022). HP-GMN: Graph Memory Networks for Heterophilous Graphs. 2022 IEEE International Conference on Data Mining (ICDM). https://doi.org/10.1109/icdm54844.2022.00165
Global-and-Local Aware Data Generation for the Class Imbalance Problem
Proceedings of the 2020 SIAM International Conference on Data Mining / Jan 01, 2020
Wang, W., Wang, S., Fan, W., Liu, Z., & Tang, J. (2020). Global-and-Local Aware Data Generation for the Class Imbalance Problem. Proceedings of the 2020 SIAM International Conference on Data Mining, 307–315. https://doi.org/10.1137/1.9781611976236.35
Investigating and Mitigating Degree-Related Biases in Graph Convoltuional Networks
Proceedings of the 29th ACM International Conference on Information & Knowledge Management / Oct 19, 2020
Tang, X., Yao, H., Sun, Y., Wang, Y., Tang, J., Aggarwal, C., Mitra, P., & Wang, S. (2020). Investigating and Mitigating Degree-Related Biases in Graph Convoltuional Networks. Proceedings of the 29th ACM International Conference on Information & Knowledge Management. https://doi.org/10.1145/3340531.3411872
Graph Convolutional Networks with EigenPooling
Proceedings of the 25th ACM SIGKDD International Conference on Knowledge Discovery & Data Mining / Jul 25, 2019
Ma, Y., Wang, S., Aggarwal, C. C., & Tang, J. (2019). Graph Convolutional Networks with EigenPooling. Proceedings of the 25th ACM SIGKDD International Conference on Knowledge Discovery & Data Mining. https://doi.org/10.1145/3292500.3330982
Multi-dimensional Graph Convolutional Networks
Proceedings of the 2019 SIAM International Conference on Data Mining / May 06, 2019
Ma, Y., Wang, S., Aggarwal, C. C., Yin, D., & Tang, J. (2019). Multi-dimensional Graph Convolutional Networks. Proceedings of the 2019 SIAM International Conference on Data Mining, 657–665. https://doi.org/10.1137/1.9781611975673.74
Education
Arizona State University
PhD, Computer Science / July, 2018
University of Michigan
MS, Electrical Engineering: Systems / December, 2013
Shanghai Jiao Tong University
BS, Electrical and Computer Engineering / July, 2012
University of Michigan
BS, Electrical Engineering / April, 2012
Experience
Pennsylvania State University
Assistant Professor / August, 2018 — Present
Links & Social Media
Join Suhang on NotedSource!
Join Now
At NotedSource, we believe that professors, post-docs, scientists and other researchers have deep, untapped knowledge and expertise that can be leveraged to drive innovation within companies. NotedSource is committed to bridging the gap between academia and industry by providing a platform for collaboration with industry and networking with other researchers.
For industry, NotedSource identifies the right academic experts in 24 hours to help organizations build and grow. With a platform of thousands of knowledgeable PhDs, scientists, and industry experts, NotedSource makes connecting and collaborating easy.
For academic researchers such as professors, post-docs, and Ph.D.s, NotedSource provides tools to discover and connect to your colleagues with messaging and news feeds, in addition to the opportunity to be paid for your collaboration with vetted partners.
Expert Institutions
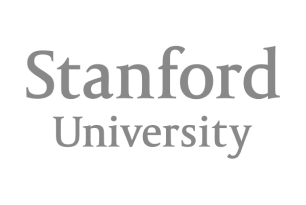
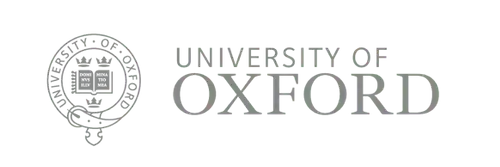
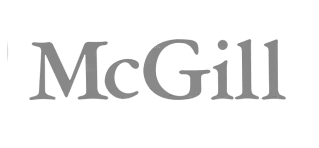
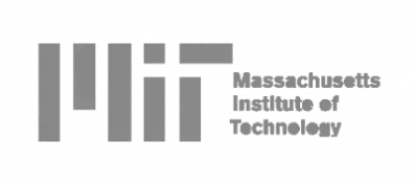
Proudly trusted by
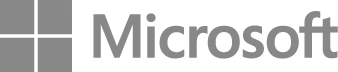

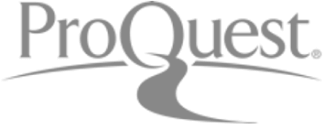
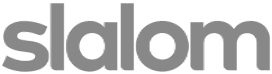

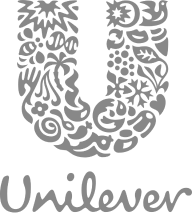